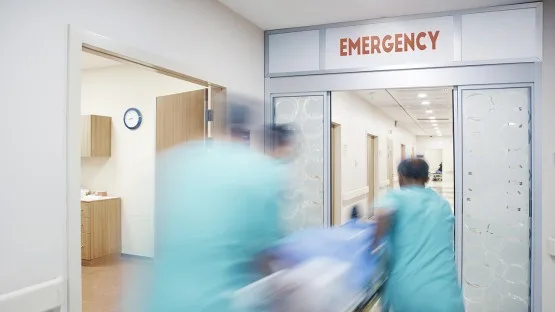
Many medical studies record a patient’s race using only the broad categories from the U.S. Census, which may conceal racial health disparities, a new study reports.
Researchers evaluated the performance of clinical risk scores for people of different races in an emergency department (ED). A clinical risk score summarizes the severity of a patient’s symptoms into a single number – the higher the score, the greater the risk of severe health outcomes. The researchers looked at broad race categories, like “Asian,” and more specific subgroups, like “Chinese” and “Indian.” The risk scores performed much better for some subgroups than others, but lumping groups together under broad race categories hid those differences.
“Any time you have a predictive risk score that works better for some groups than for other groups, that’s potentially a problem, because it means that the errors in that risk score may have a bigger impact on some groups than on others,” said senior author Emma Pierson, assistant professor of computer science in the Jacobs Technion-Cornell Institute at Cornell Tech, and in the Cornell Ann S. Bowers College of Computing and Information Science.
Often, there were greater differences among subgroups within the same race category than between the broad race categories themselves. Clinical risk scores can influence what kind of care a patient receives, and so performance differences could cause some groups to be systematically denied care.
“To the best of our knowledge, we present the first analysis of disparities in risk scores in health care that looks at this granular level,” said Rajiv Movva, a doctoral student in the field of computer science and co-first author on the study. “Optimistically, if you do this more granular analysis, you can better describe your population and also think more carefully about possible interventions.”
Movva presented the study, “Coarse race data conceals disparities in clinical risk score model performance,” on Aug. 11 at the Machine Learning for Healthcare 2023 meeting.
In the new study, researchers looked at two commonly used clinical risk scores for triaging the most at-risk patients. They also used patient demographic and health information to develop their own risk scores using machine learning to predict a patient’s risk of hospitalization, death or transfer to the ICU, or a quick return to the emergency room after discharge. The data came from an existing dataset of about 418,000 emergency room visits to a Boston hospital.
When it came to risk score performance, some subgroups were consistently outliers. Brazilians and Russians in the white group; Africans, Caribbeans and Cape Verdeans in the Black group; and Koreans and Southeast Asians in the Asian group all had risk score results that often differed from the group averages.
The researchers also found evidence of the “healthy immigrant effect” – an observation that immigrants often have better health than people with a similar background who were born in the U.S. For example, people who identified as “Black” had more health problems than people who identified as “Black-African” or “Black-Caribbean,” and thus may have been more likely to be immigrants.
Disparities in how the risk scores performed for the subgroups could be traced to different patterns of symptoms and health outcomes. One reason for these differences may be social and environmental factors the subgroups share, the researchers said.
While this study focused on emergency visits, the researchers expect this phenomenon occurs across the field of algorithmic fairness – the study of understanding and correcting biases in computer models – which historically has used broad race categories.
“The findings suggest that there is much more work to be done in terms of examining performance disparities across groups when we have better descriptions of the groups themselves,” said Divya Shanmugam, co-first author and doctoral student at the Massachusetts Institute of Technology.
Nikhil Garg, assistant professor of Operations Research and Information Engineering at Cornell Engineering and at the Jacobs Technion-Cornell Institute at Cornell Tech; Kaihua Hou, doctoral student at the University of California, Berkeley; Priya Pathak, assistant professor of pediatrics at Columbia University and John Guttag, professor of computer science and electrical engineering at MIT, contributed to the study. Pierson also has an appointment with Weill Cornell Medicine.
This research was supported by a Google Research Scholar award, an NSF CAREER award, a CIFAR Azrieli Global scholarship, a LinkedIn research award, Wistron Corporation, a Future Fund regrant, a Meta research award, a Cornell Tech Urban Tech Hub grant and an NSF Graduate Research Fellowship Program award.
Patricia Waldron is a writer for the Cornell Ann S. Bowers College of Computing and Information Science.